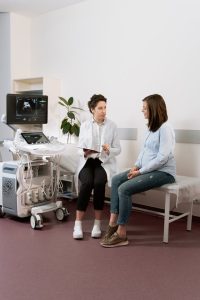
© Pexels/Mart Production
For healthcare professionals, it can be difficult to assess the risk of PTB because risk factors can vary from one woman to another. Women who have already been pregnant (parous women) have different and additional risk factors than those in their first pregnancy (nulliparous women). In addition, there are individual aspects to consider. Therefore, machine learning models can serve as screening tools and help clinicians to assess the individual woman’s risk factors, even if those are not so common and obvious. In the present study, six different machine learning models were tested to predict the risk of PTB in a cohort of 3,509 pregnant women in the United Arab Emirates. The artificial intelligence detected the most prevalent risk factors for parous and nulliparous women, including higher maternal age, preeclampsia, placenta previa, BMI at delivery, and amniotic fluid infection. The study concludes that machine learning models have the potential to become a great support in the future risk prediction of PTB.
The causes of preterm birth are complex and multifaceted and can affect outcomes significantly. PTB is, for example, still a leading cause of mortality and morbidity in childhood. Healthcare professionals must refer to an extensive list of risk factors to determine how likely a PTB is for each individual patient. These risk factors include advanced maternal age, smoking, substance abuse, economic factors, obstetric history, and other pregnancy-related conditions. Unfortunately, these symptoms are very common and nonspecific for PTB. The question remains as to which risk factors have the greatest influence on PTB and whether they can be used to predict PTB. There are already some assessment methods for predicting a woman’s risk for PTB; however, these are often not reliable and practicable. The statistical models are usually limited to single risk factors and do not fully cover the complexity of PTB. Substance abuse, for example, can cause a PTB but also a host of other pregnancy complications. In addition, these models lack the interpretability required for everyday clinical use. The present study aims to address these issues by comparing six different machine learning (ML) models in their ability to predict PTB. ML is a type of artificial intelligence that can train itself with the help of data in order to make ever better predictions of the mothers’ risk factors. 3,509 women from the Mutaba’ ah study in the United Arab Emirates were included in the detailed assessment of the mothers’ risk factors for PTB.
PTB risk factors differ greatly between parous and nulliparous women
The assessment revealed that the five most influential characteristics for PTB in parous women are higher maternal age, previous PTBs, previous caesarean sections, preeclampsia, and placenta previa. Other risk factors included exposure to passive smoking, a history of infertility treatments, higher gravidity, lower educational levels, preexisting hypertension, diabetes mellitus, a previous pregnancy loss, BMI at delivery, and interpregnancy interval. In addition, mothers suffering from antepartum haemorrhage, oligohydramnios, infection of the amniotic sac, and placental disorders, Streptococcus carrier B, or genitourinary infection were at higher risk to deliver preterm. The analysed risk factors differed significantly in nulliparous mothers. In these women, previous PTB and caesarean delivery could be excluded. Instead, BMI at delivery, maternal age, amniotic fluid infection, premature rupture of membranes, and preeclampsia were identified as the most influential risk factors. Other influences may include physical activity, diabetes, oligohydramnios, and a history of infertility treatment.
ML models show potential to predict an individual’s risk for PTB
The ML models were used to generate an individual risk factor for each pregnant woman. Of the six models evaluated, XGBoost showed the best performance in predicting the risk of PTB. The models are characterized by their ability to learn from new inputs and identify the most important influences. In the future, the ML models can be used as screening tool to detect risks earlier and thus improve health outcomes. They increase the accuracy of disease prediction and support diagnosis. Furthermore, they have the potential to improve the patient experience and reduce the economic burden on health systems. In practice, the models can help healthcare professionals make informed decisions. If the ML model classifies a patient as being at-risk, their treatment and monitoring can be adapted. This is particularly valuable in the risk prediction for asymptomatic and nulliparous women. In addition, XGBoost is also recommended as ML model of choice to predict other diseases.
Paper available at: https://journals.plos.org/plosone/article?id=10.1371/journal.pone.0293925
Ful list of authors: Wasif Khan, Nazar Zaki, Nadirah Ghenimi, Amir Ahmad, Jiang Bian, Mohammad M. Masud, Nasloon Ali, Romona Govender, Luai A. Ahmed
DOI: https://doi.org/10.1371/journal.pone.0293925
More information on preeclampsia and other risk factors of PTB: https://www.efcni.org/health-topics/pregnancy/pregnancy-complications/#3